Digital Marketing Statistics That Will Shape 2023
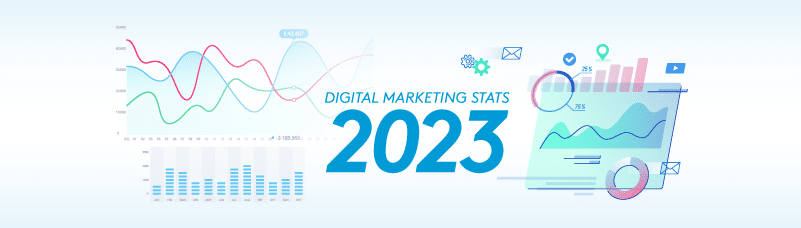
Where is the state of digital advertising post-pandemic and in the midst of economic concerns?
Digital advertising growth is slowing down from immediate post-pandemic years, but it is not decreasing dramatically. Instead, it is leveling off towards a more moderate pace.
In 2021, the growth rate for U.S. digital ad spend reached 35 percent – the highest ad spend increase since 2006. The following year, 2022, saw continued growth with an increase of 20 percent. Fast-forward to 2023, digital marketing statistics show that U.S. ad spend will have a smaller increase of 13.9 percent, reaching $297.4 billion by the end of the year compared to $261.1 billion in 2022.
The digital advertising industry has shown resilience in the past and adapted to changes in the market. That looks to continue as the growth of ecommerce and consumers’ shifting behavior will result in pivoting ad budgets towards affiliate marketing and other digital channels in 2023.
Digital marketing statistics to grow your business
In this post, CAKE has compiled a list of noteworthy digital marketing statistics to help you leverage data to guide your marketing strategy, successfully hit those 2023 KPIs, and grow your business.
Affiliate marketing
Affiliate marketing has seen sustainable growth in recent years, and this trend is expected to continue with the ongoing evolution of digital marketing and ecommerce.
The multibillion-dollar industry’s upward trend accelerated during COVID-19 and remained strong post-pandemic because of marketers’ prioritization of a pay-per-performance approach. In fact, the affiliate industry grew 47 percent between 2018 and the end of 2021. With that, the global affiliate marketing market size is expected to expand at a CAGR of 9.89 percent reaching $27.78 billion by 2027.
- Globally, the affiliate marketing industry is currently valued at $17 billion. (Demandsage)
- Interest in affiliate marketing has steadily increased since 2016, reaching its peak in January 2023. (Google Trends)
- In a recent report, 80 percent of respondents view affiliate partnerships as more important revenue-wise in 2023 compared to previous years and are allocating more budget toward the channel. (PMA)
- The affiliate marketing industry is comprised of 96,660 affiliate networks worldwide. (Datanyze)
- The vast majority of advertisers – 83 percent – use affiliate networks to secure deals with publishers. (Forrester Group)
- Affiliate marketing programs generate 15-30 percent of all sales for advertisers. (Authority Hacker)
- Marketers turn to the affiliate industry to boost their brand awareness (83 percent) and engage with existing customers (79 percent). (Rakuten)
Social
- Social media global ad spend is expected to reach $268.7 billion in 2023. (Statista)
- As a result of Apple’s privacy changes, social media ad spend growth is projected to hit its lowest annual growth to date, 7.5 percent. (Forbes)
- In the U.S., social media ad spend is projected to reach $94.4 billion in 2023. (Statista)
- There are 4.89 billion social media users worldwide in 2023, a 6.5 percent increase year-over-year. (Statista)
- 90.4 percent of millennials report using social media, compared to 77.5 percent of Gen X, and 48.2 percent of baby boomers. (eMarketer)
- 55 percent of individuals purchase products online after discovering them on social media. (Kleiner Perkins)
- 70 percent of social media marketers believe Facebook is the most useful platform for meeting their goals. (Sprout Social)
- Nearly 40 percent of people 18 to 24 are turning to visual-based social media platforms like TikTok or Instagram for their internet searches. (TechCrunch)
Search
- In 2022, search ad spend stood at $164.6 billion worldwide. This amount is projected to grow to roughly $190.5 billion in 2024. (Statista)
- 75 percent of people say paid search ads make it easier to find information that they’re looking for. (Clutch)
- Google’s responsive search ads are becoming increasingly popular. More than 84 percent of advertisers are using this ad type or plan to use it in 2023. (Demandsage)
- Long-tail keywords make up more than 70 percent of all web searches, and typically garner a 36 percent conversion rate. (Search Engine Watch)
- The average cost per action (CPA) is $49 for paid search. (WordStream)
- The average click-through rate (CTR) is 3.17 percent for paid search. (WordStream)
- Appearing in mobile search ad results can boost brand awareness by 46 percent. (Think with Google)
Ecommerce
- U.S. retail ecommerce revenue is predicted to reach $1.1 trillion in 2023, a 21.5 percent increase from 2022. (Statista)
- U.S. online sales climbed from $257 billion for the 2021 holiday season to $270 billion for 2022, in contrast to global holiday sales, which were flat compared to last year at $1.14 trillion. (Forbes)
- 218.8 million U.S. consumers will shop online in 2023, which is about 65 percent of the U.S. population. (Oberlo)
- In 2023, ecommerce sales are forecasted to account for 20.8 percent of worldwide retail sales. (Oberlo)
- Merchants see an average of 23 percent of their revenues coming from affiliate marketing, which is on par with SEO and PPC marketing. (Authority Hacker)
- The average online ecommerce order is 31 percent higher with affiliate marketing customers. (CJ Affiliates)
- Consumers are likely to spend 47 percent more when they have a personalized experience. (e-commerce Platforms)
For more ecommerce statistics, check out our blog here.
Video
- Video advertising is projected to reach $210.2 billion in ad spend in 2023. (Statista)
- Video ad spend is forecasted to show a CAGR of 10.97 percent. (Statista)
- This year, advertisers will spend $28.21 billion on social media video advertising. (Statista)
- Mobile and desktop video ad spend is expected to show an annual growth rate of 5.1 percent between 2021-2025. (Statista)
- People are 1.5x more likely to watch video daily on a smartphone than on a computer. (Facebook)
- 83 percent of video marketers say video has helped them generate leads and 84 percent of people say they’ve been convinced to buy a product or service after watching a brand’s video. (Wyzowl)
- Approximately 86 percent of marketers are using video content in their campaign outreach efforts. (IAB)
- 82 percent of internet traffic will come from video by 2022. (Cisco)
- In 2023, email marketing revenue is set to cross the $10-billion mark. (Statista)
- 31 percent of B2B marketers say email newsletters are the best way to nurture leads. (Content Marketing Institute)
- Affiliate marketing and email marketing account for 16 percent of all ecommerce transactions in the U.S. and Canada. (Business Insider)
- For every $1 spent on email marketing, the average campaign earns $42 in ROI. (DMA, 2019)
- Segmented email campaigns result in a 760 percent increase in revenue. (Campaign Monitor)
- Emails that contain personalized subject lines are 26 percent more likely to be opened. (Campaign Monitor)
Mobile
- Global mobile ad spend is expected to increase by 7.5 percent and reach $362 billion in 2023. (App Annie)
- In 2023, U.S. mobile ad spend will increase 14.04 percent to $194.8 billion. (eMarketer)
- Economic factors and privacy regulations are set to dampen mobile gaming spend in 2023, which is expected to drop to $107 billion in 2023. (App Annie)
- Almost 25 percent of companies invest in mobile optimization as a top SEO tactic. (HubSpot)
- 80 percent of users are more likely to purchase from companies with mobile sites or apps. However, 57 percent of mobile users will not recommend a business with a poorly designed mobile site. (Think with Google)
- More than 50 percent of online U.S. traffic originates from mobile devices. (Statista)
- On average 53 percent of emails are opened on mobile devices. (Campaign Monitor)
Display
- U.S. display advertising is expected to increase 15.5 percent in 2023, reaching $165.84 billion. This growth rate is 5.4 percent less compared to 2022. (eMarketer)
- In 2023, U.S. display advertising will account for 43.8 percent of media spending. (eMarketer)
- The Google Display Network reaches 90 percent of internet users worldwide. (Google)
- Customers are 155 percent more likely to search for brand-specific terms when exposed to display ads. (EMSC)
- The average CPA is $76 for display ads. (WordStream)
- Advertisers who leverage smart display campaigns have 20 percent more conversions at the same CPA when evaluated against traditional display campaigns. (Google)
Other content you might be interested in:
- Blog: How to Start an Affiliate Marketing Program – 5 Tried-and-True Tips
- Blog: Brand Integrity and Affiliate Marketing – 6 Affiliate Marketing Pitfalls and How to Avoid Them
- Blog: 6 Signs To Know If You’re Using The Right Affiliate Network Software